Note
Go to the end to download the full example code
Plot types#
This example shows how to start an Ansys Dynamic Reporting service via a Docker image and create different plot items. The example focuses on showing how to use the API to generate different plot types.
Note
This example assumes that you do not have a local Ansys installation but are starting an Ansys Dynamic Reporting Service via a Docker image on a new database.
Start an Ansys Dynamic Reporting service#
Start an Ansys Dynamic Reporting service via a Docker image on a new database. The path for the database directory must be to an empty directory.
import numpy as np
import ansys.dynamicreporting.core as adr
db_dir = r"C:\tmp\new_database"
adr_service = adr.Service(ansys_installation="docker", db_directory=db_dir)
session_guid = adr_service.start(create_db=True)
Create a simple table#
Let us start by creating a simple table and visualizing it. Create a table with 5 columns and 3 rows.
simple_table = adr_service.create_item(obj_name="Simple Table", source="Documentation")
simple_table.item_table = np.array(
[[0, 1, 2, 3, 4], [0, 3, 6, 9, 12], [0, 1, 4, 9, 16]], dtype="|S20"
)
simple_table.labels_row = ["X", "line", "square"]
You can use the labels_row attribute to set the row labels. Use the visualize method on the object to see its representation. By default, it will be displayed as a table
simple_table.visualize()
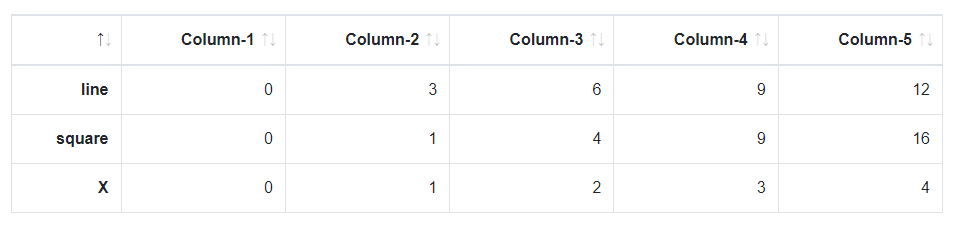
Visualize as a line plot#
Let us know create a new item that is the same as the previous simple table, but this time we will set the plot attribute to line to visualize the values as two line plots, and we will use the xaxis attribute to set which row should be used as the X axis. We can also control the formatting and the title of the axis separately with the *axis_format and *title attributes, as done below. The result can be seen in the following image.
line_plot = adr_service.create_item(obj_name="Line Plot", source="Documentation")
line_plot.item_table = np.array([[0, 1, 2, 3, 4], [0, 3, 6, 9, 12], [0, 1, 4, 9, 16]], dtype="|S20")
line_plot.labels_row = ["X", "line", "square"]
line_plot.plot = "line"
line_plot.xaxis = "X"
line_plot.yaxis_format = "floatdot0"
line_plot.xaxis_format = "floatdot1"
line_plot.xtitle = "x"
line_plot.ytitle = "f(x)"
line_plot.visualize()
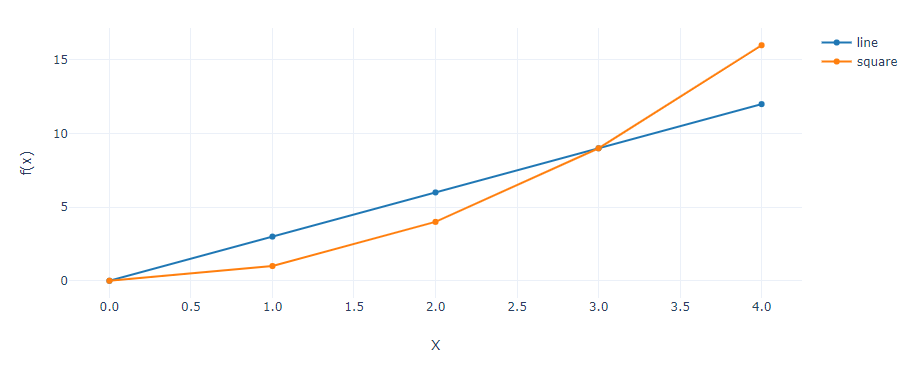
Visualize as a bar plot#
Next, we will see how to create a bar plot, and decorate it with the same attributes used in the previous code snippet. See the following image for the resulting visualization.
bar_plot = adr_service.create_item(obj_name="Bar Plot", source="Documentation")
bar_plot.item_table = np.array([[0, 1, 2, 3, 4], [0.3, 0.5, 0.7, 0.6, 0.3]], dtype="|S20")
bar_plot.plot = "bar"
bar_plot.labels_row = ["ics", "my variable"]
bar_plot.xaxis_format = "floatdot0"
bar_plot.yaxis_format = "floatdot2"
bar_plot.xaxis = "ics"
bar_plot.yaxis = "my variable"
bar_plot.visualize()
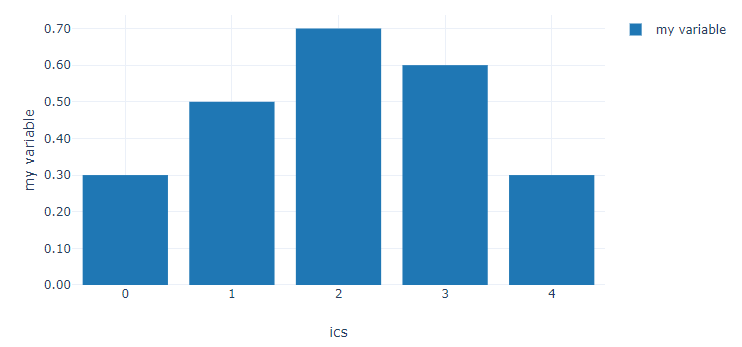
Visualize a pie chart#
Next supported plot type is the pie chart. Please see the following code snippet to generate the pie chart as in the following image.
pie_plot = adr_service.create_item(obj_name="Pie Plot", source="Documentation")
pie_plot.item_table = np.array([[10, 20, 50, 20]], dtype="|S20")
pie_plot.plot = "pie"
pie_plot.labels_column = ["Bar", "Triangle", "Quad", "Penta"]
pie_plot.visualize()
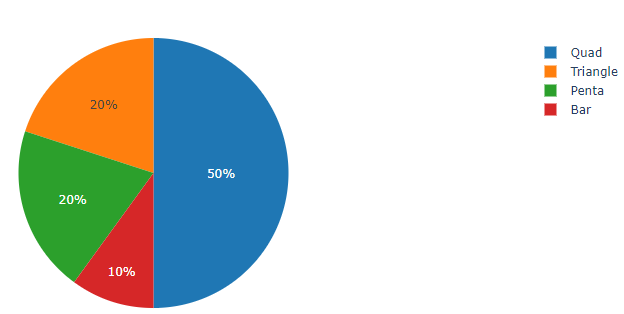
Visualize a heatmap#
Heatmaps are plots where at each (X,Y) position is associated the value of a variable, colored according to a legend. Here the snippet on how to create a heatmap representation - please note how nan values are also supported, resulting in empty cells.
heatmap = adr_service.create_item(obj_name="Heatmap", source="Documentation")
heatmap.item_table = np.array(
[
[0.00291, 0.01306, 0.02153, 0.01306, 0.00291],
[0.01306, 0.05854, 0.09653, 0.05854, 0.01306],
[0.02153, 0.09653, np.nan, 0.09653, 0.02153],
[0.01306, 0.05854, 0.09653, 0.05854, 0.01306],
[0.00291, 0.01306, 0.02153, 0.01306, 0.00291],
],
dtype="|S20",
)
heatmap.plot = "heatmap"
heatmap.format = "floatdot0"
heatmap.visualize()
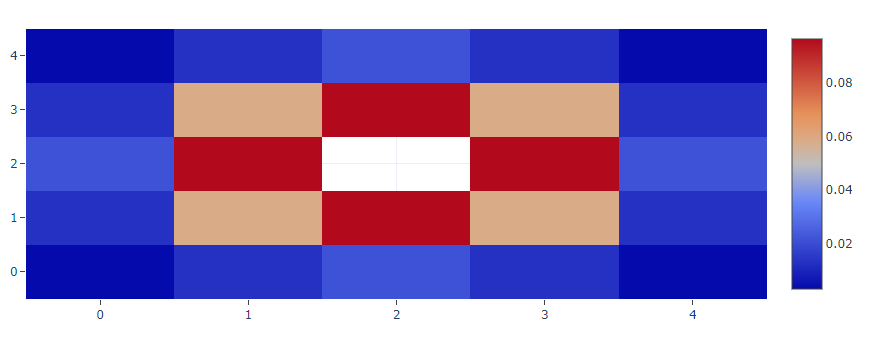
Visualize a parallel coordinate plot#
Parallel coordinate plots are especially useful when analyzing data coming from multiple runs. Place in each raw the values of variables for a given simulation. Each column is a different variable. The parallel coordinate plot allows you to visualize all this data in a way that stresses correlations between variables and runs.
parallel = adr_service.create_item()
parallel.item_table = np.array(
[
[54.2, 12.3, 1.45e5],
[72.3, 9.3, 4.34e5],
[45.4, 10.8, 8.45e4],
[67.4, 12.2, 2.56e5],
[44.8, 13.5, 9.87e4],
],
dtype="|S20",
)
parallel.labels_column = ["Temperature", "Max. Pressure", "Max. Work"]
parallel.plot = "parallel"
parallel.visualize()
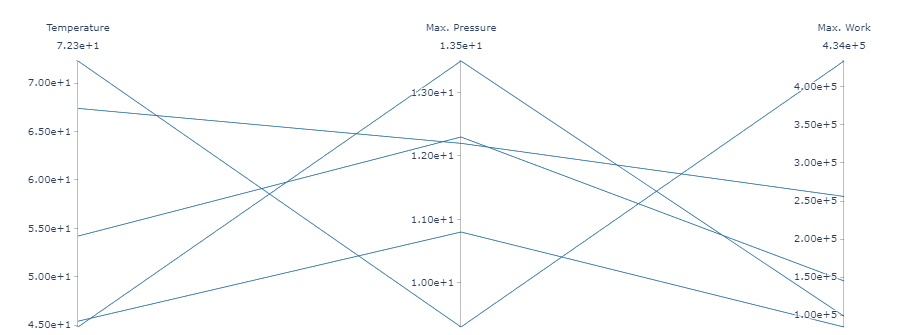
Visualize a Sankey diagram#
A Sankey diagram allows you to visualize the relationship between different elements. For this reprenstation, place the information inside a squared table.
sankey_plot = adr_service.create_item()
sankey_plot.item_table = np.array(
[
[0, 0, 8, 2, 0, 0],
[0, 0, 0, 4, 0, 0],
[0, 0, 0, 0, 8, 0],
[0, 0, 0, 0, 5, 1],
[0, 0, 0, 0, 0, 0],
[0, 0, 0, 0, 0, 0],
],
dtype="|S20",
)
sankey_plot.labels_row = ["A", "B", "C", "D", "E", "F"]
sankey_plot.labels_column = ["A", "B", "C", "D", "E", "F"]
sankey_plot.plot = "sankey"
sankey_plot.visualize()
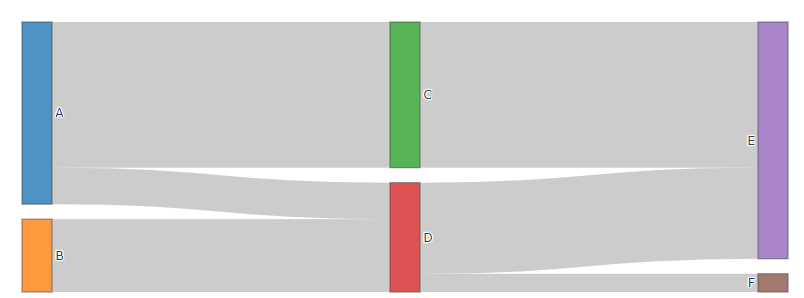
Close the service#
Close the Ansys Dynamic Reporting service. The database with the items that were created remains on disk.
# sphinx_gallery_thumbnail_path = '_static/01_connect_3.png'
adr_service.stop()
Total running time of the script: (0 minutes 0.000 seconds)